Why 95% Confidence Interval?
Ever wondered why we're so hung up on this 95% confidence interval thing? Let's dive in and figure it out together.
The 95% Confidence Interval: What's the Big Deal?
First off, let's get real. The 95% confidence interval isn't some magic number that fell from the sky. It's just become the go-to standard in a lot of fields, especially when we're talking about experimentation and statistical significance.
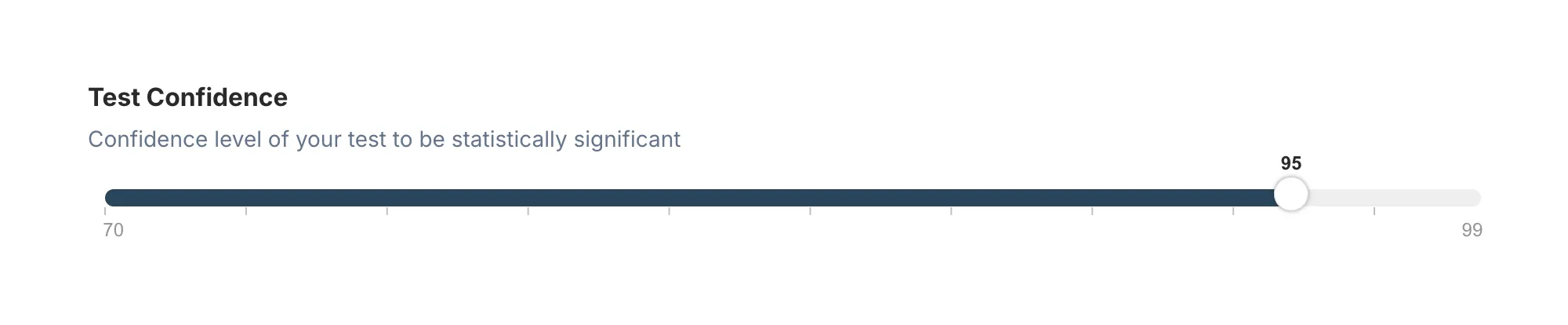
But why 95%? Why not 90% or 99%? Well, it's a bit of a balancing act.
The Trade-off Game
Here's the deal: when we're running experiments, we're always playing a game of trade-offs. We want to be confident in our results, but we also don't want to make it impossibly hard to find significant effects.
Type 1 Error: Too low (like 80%), and we risk too many false positives.
Type 2 Error: Too high (like 99%), and we might miss out on real effects (false negatives).
The 95% level kind of hits a sweet spot. It's stringent enough to give us some confidence, but not so strict that we never find anything interesting.
A Bit of History
Believe it or not, this 95% thing has been around for a while. Ronald Fisher, a big shot in statistics, kind of set the stage back in the 1920s. He suggested using the 5% significance level, which is the flip side of our 95% confidence interval.
Fisher wasn't saying it was the only way to go, but it caught on. It became a convention, like how we shake hands instead of bowing or whatever.
What Does 95% Confidence Really Mean?
Okay, let's break this down. When we say we have a 95% confidence interval, we're saying:
"If we ran this experiment a bunch of times, about 95% of the time, our true population parameter would fall within this interval."
It's not saying there's a 95% chance our result is right. It's more about the process than any single result.
The P-value Connection
You've probably heard about p-values too. They're closely related to confidence intervals. A 95% confidence interval is basically saying, "These are all the values that would give us a p-value lesser than 0.05."
Why Not Always Go Higher?
You might be thinking, "Why not always use 99% or even higher?" Well, there are a few reasons:
- Sample Size: Higher confidence needs bigger sample sizes. That means more time, more money, more everything.
- Practical Significance: Sometimes, a 95% interval is good enough for making decisions. Do we really need to be 99% sure before we change a button color?
- False Negatives: The higher we set our bar, the more likely we are to miss real effects.
Real-World Application
Let's say you're running an A/B test on your website. You're comparing two versions of a landing page. After running the test, you get a 95% confidence interval for the difference in conversion rates of 2% to 8%.
What does this mean in plain English? It means you can be pretty confident (95% confident, to be exact) that if you implemented the new version, your true increase in conversions would be somewhere between 2% and 8%.
Is that good enough to make a decision? Maybe. It depends on your risk-benefit profile and what kind of changes you're making.
The Controversy
Now, don't think everyone's on board with this 95% thing. There's been plenty of debate.
Andrew Gelman, a stats guru, has argued for using 50% intervals instead. His point? It gives a better sense of uncertainty and prevents people from treating the 95% interval as a hard cutoff.
Others have pointed out that people often misinterpret confidence intervals, thinking they're more definitive than they really are.
Alternatives and Considerations
While 95% is standard, it's not the only game in town:
- 90% Confidence: Used when you're okay with a bit more uncertainty.
- 99% Confidence: When you really need to be sure (like in medical trials).
- Bayesian Methods: A whole different approach that some argue is more intuitive.
The Bottom Line
The 95% confidence interval isn't perfect, but it's a useful tool. It gives us a standardized way to talk about uncertainty in our results.
The key is understanding what it really means and using it as part of a broader decision-making process. It's not the be-all and end-all, but a piece of the puzzle.
FAQs
Q: Is 95% confidence always the best choice?
A: Not always. It depends on your specific needs and the consequences of being wrong.
Q: Can I use different confidence levels for different tests?
A: Absolutely. Just be clear about what you're using and why.
Q: Does a 95% confidence interval mean I'm 95% sure of my result?
A: Not quite. It's about the process, not any single result.
Q: How does sample size affect the confidence interval?
A: Generally, larger sample sizes lead to narrower confidence intervals.
Q: Should I always aim for statistical significance before making decisions?
A: Not necessarily. Sometimes practical significance is more important than statistical significance.
Remember, stats are tools, not rules. Use them wisely, and they'll serve you well in your experimentation journey.