What Is Peeking in A/B Testing and Why You Shouldn’t Peek!
A/B testing is an incredibly powerful tool for optimizing your website or app. However, to get reliable insights, following proper testing methodologies is crucial. One common mistake that can compromise the validity of your results is peeking.
In this blog post, we’ll explore what peeking in A/B testing is, why it’s tempting, and most importantly, why you should avoid it at all costs.
What Is Peeking in A/B Testing?
Peeking refers to checking the results of an A/B test before it has reached its predetermined sample size or completed its intended duration. Imagine conducting an experiment where you flip a coin 100 times to test whether it’s biased. If you stop and analyze the results after just 10 flips, you risk drawing conclusions based on randomness rather than true probability.
Similarly, in A/B testing, peeking disrupts the statistical integrity of your test by increasing the likelihood of false positives or negatives.
Why Peeking Happens
Business Pressures
With deadlines approaching, stakeholders eager for updates, and decisions needing to be made quickly, the temptation to check test results prematurely can be hard to resist.
Psychological Factors
On a personal level, curiosity can drive testers to peek. Early test results might show dramatic differences, feeding into the desire for quick wins or validation.
There’s also the fear of missing out (FOMO)—what if one variant is clearly outperforming the other, and you’re delaying potential gains?
These pressures and biases make it difficult to wait until the test is truly ready for analysis.
The Statistical Impact of Peeking
Peeking isn’t just a procedural error—it’s a statistical one. Here’s how it compromises your test:
The Multiple Testing Problem
Every time you peek at your results, you’re essentially conducting a new statistical test. This increases the likelihood of finding a “false positive” result (Type I error). If your confidence level is set at 95%, there’s already a 5% chance of observing a false positive. Peeking five times can raise this chance significantly, invalidating your findings.
Type I Error Inflation
When you analyze data repeatedly, the cumulative probability of error grows. This means you might declare a winner prematurely based on random fluctuations rather than true performance differences.
For example, if you peek at your test results after 20%, 40%, and 60% of your sample size, the statistical significance calculations are no longer valid. What seems like a “clear winner” may revert to average once the full dataset is collected.
Traditional Solutions to Avoid Peeking
Historically, A/B testing frameworks have relied on rigid methodologies to prevent peeking.
Fixed-Horizon Testing
This approach requires you to define:
- A fixed sample size
- A predetermined test duration
- A strict no-peeking rule until the test is complete
While this method is statistically robust, it lacks flexibility. If one variant performs significantly better early on, you still have to wait until the test concludes, which can be inefficient.
Bonferroni Correction
This statistical adjustment reduces the risk of false positives when multiple comparisons are made. While effective, it can be overly conservative, leading to longer test durations and less actionable insights in the short term.
Modern Solutions: Sequential Testing
What Is Sequential Testing?
Sequential testing offers a more dynamic alternative to traditional methods. It allows for continuous monitoring of test results without the statistical penalties associated with peeking.
Unlike fixed-horizon testing, sequential testing adjusts significance thresholds dynamically, ensuring that statistical rigor is maintained even when data is reviewed multiple times.
How It Works
- Sequential testing evaluates data in real-time as it’s collected.
- Significance thresholds are updated dynamically to account for multiple looks at the data.
- Automated stopping rules determine when enough evidence has been gathered to declare a winner.
This approach allows for flexibility while preserving the validity of your results.
Why Sequential Testing Is a Game Changer
1. Faster Decision-Making
If there’s a clear winner early on, sequential testing can end the experiment sooner, saving time and resources.
2. No Fixed Sample Size
You don’t need to calculate a rigid sample size in advance. Sequential testing adapts dynamically to the data, making it more efficient for real-world scenarios.
3. Preserves Statistical Integrity
By accounting for multiple peeks at the data, sequential testing ensures that your error rate remains controlled, providing reliable results even with continuous monitoring.
How Mida Helps You Avoid Peeking
Mida’s A/B testing platform incorporates modern statistical techniques like sequential testing to eliminate the risks of peeking. With real-time monitoring, automated stopping rules, and built-in significance calculations, Mida empowers you to make faster, data-backed decisions without compromising on accuracy.
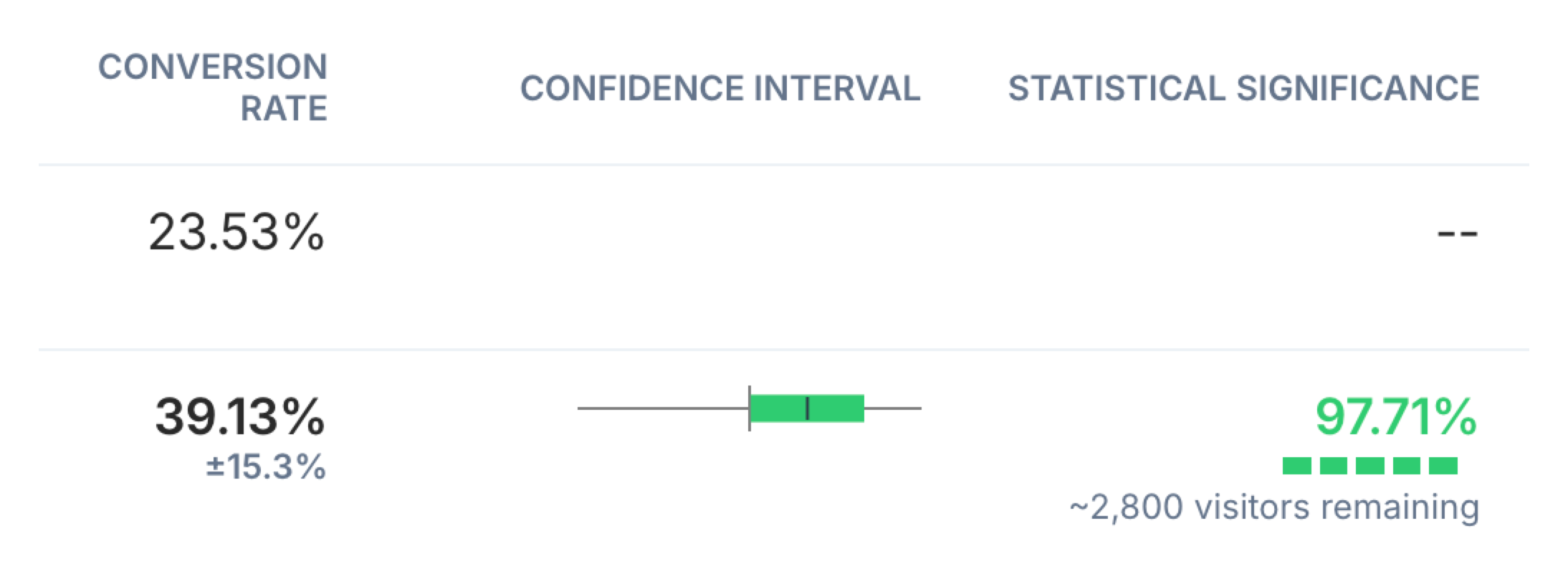
Key features include:
- Real-time analytics without statistical penalties
- Dynamic sample size adjustments
- Automatic notifications when a test reaches significance
By using tools like Mida, you can focus on driving results instead of worrying about statistical pitfalls.
Final Thoughts
Peeking may seem like a minor misstep, but its impact on your A/B test results can be profound. Traditional methods like fixed-horizon testing discourage peeking but lack flexibility, while modern approaches like sequential testing offer a more agile and reliable solution.
Whether you’re new to A/B testing or a seasoned professional, understanding the risks of peeking and leveraging tools like Mida will help you run better experiments, faster.
Ready to optimize smarter? Try Mida for free today and take the guesswork out of your A/B testing.