What are Type 1 and Type 2 Errors?
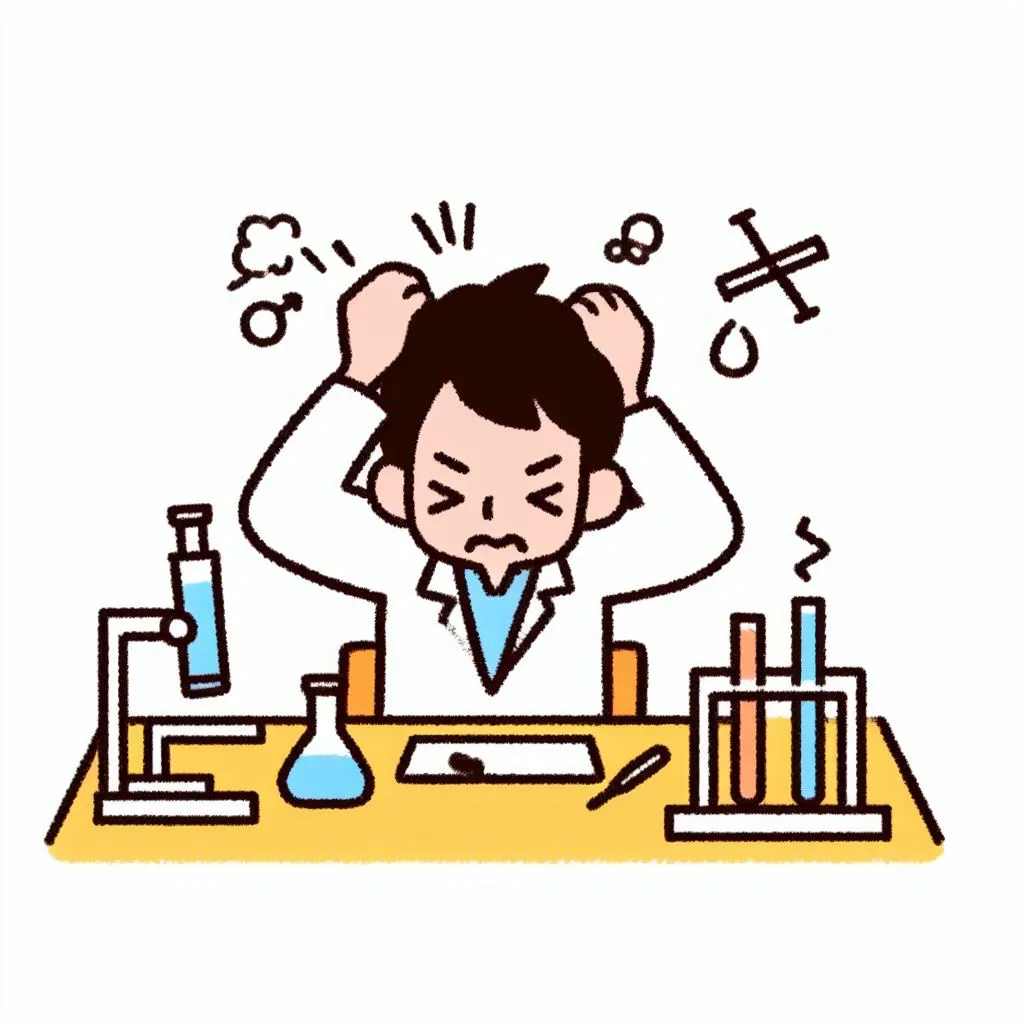
Type I and Type II errors occur when our experimental conclusions either falsely identify a successful outcome or overlook a genuine one.
In both cases, the decisions are influenced by perceived rather than actual results. Now, why is this important to understand?
These errors are central to hypothesis testing in statistics. Lacking an understanding of them can lead to wrong conclusions or decisions from test results.
Let’s understand these terms a bit:
- Type I Error: Otherwise known as a false positive. This happens when you conclude something has an impact but in reality, it doesn’t.
- Type II Error: Known as a false negative. This occurs when you conclude that something doesn’t affect the outcome while in reality, it does.
Why Do These Errors Occur?
A bit of theory! In A/B testing, you have a null hypothesis (H0) and an alternative hypothesis (H1).
- Null hypothesis (H0): The new website design has no impact on the conversion rate
- Alternative hypothesis (H1): The new website design impacts the conversion rate
So, what we essentially do in A/B testing is trying to decide whether to accept the H1 and reject the H0.
But as we humans are not perfect so are our experiments and conclusions. This is where errors creep in – Type I and Type II.
Type I error – Alpha (α)
Type I error, also known as false positives is denoted by alpha (α) the significance level.
Let’s say you run an experiment to see if a new green ‘buy’ button increases conversions as opposed to a red one. You conclude the green button increases conversions. But, this result occurred purely by chance and not because the button was better. This is a Type I error.
The chance of this error happening is what we call the significance level or alpha, often set to 5%. Simply put, we’re willing to accept a 5% chance we’re wrong when we declare a winner.
Type II error – Beta (β)
Type II error, also known as false negatives is denoted by beta (β). It occurs when you fail to realize that there’s an effect when there actually is.
Continuing from the previous example, let’s say the green ‘buy’ button really does increase conversions. But, your experiment concludes that it doesn’t. This is a Type II error.
The risk of committing a Type II error is inhibited by the statistical power of a study. In general, a power level of 80% or more is considered acceptable.

The Trade-Off
The tricky part is, these two types of errors are inversely related. Reduce your chance of a Type I error, the chance of a Type II error increases, and vice versa.
It’s a fine balance you have to maintain between the two. The ideal is to minimize both errors but often that’s not feasible. You then rely on the context to decide which error could be more detrimental.
Why does it matter in A/B testing?
A/B testing relies heavily on statistical decisions. Having an understanding of these errors helps understand the results more effectively. It streamlines the decision-making process.
Remember, Type I and II errors cannot be completely avoided. The main idea is to manage and minimize them.
We must bear in mind that these errors occur, accept them, and more importantly, know how to handle them. By doing so, we can make more accurate and reliable decisions.
Complicated as they may sound, understanding Type I and Type II errors are truly an integral part of the optimization journey.
So, remember to keep Type I and II errors under check, and happy testing!