Frequentist vs Bayesian in A/B Testing
There is a long-standing discourse in A/B testing that revolves around philosophical statistics - the Bayesian against the Frequentist approach.
This debate is not a recent development. In fact, its origins date back to the 18th century when Thomas Bayes penned a significant essay named “An Essay towards solving a Problem in the Doctrine of Chances”. This contentious issue remains quite relevant to modern CRO, where some tools gravitate towards Bayesia while others use Frequentist algorithm.
Understanding these two methodologies can serve as a significant asset for your next A/B testing experiments. However, understanding the difference between the two can be very confusing.
In this article, instead of diving deep into mathematical equations, we’ll use a simple coin-toss experiment to understand these methodologies. In this experiment, we’ll flip a coin a certain number of times and document how many times it lands on heads.
Frequentist Statistics

The frequentist approach in a coin-toss experiment is a theory of probability that focuses on the frequency of an event occurring in repeated trials. In this approach, the probability of an event is understood as the long-run relative frequency of its occurrence when the experiment is repeated an infinite number of times.
For example, let's consider flipping a fair coin. The frequentist approach would state that the probability of getting heads is 0.5 because, in the long run, if we were to flip the coin many times, we would expect heads to appear approximately half the time.
In this framework, the frequency of outcomes determines our probability assessment. The more trials we conduct, the closer the relative frequency will approach the actual probability. The idea is that by conducting numerous repetitions of the experiment, we gain more confidence in our estimates of probabilities.
Overall, the frequentist approach relies on conducting repeated experiments and observing the frequencies of outcomes to estimate probabilities, treating probability as a concept associated with the long-term behavior of a process.
Bayesian Statistics
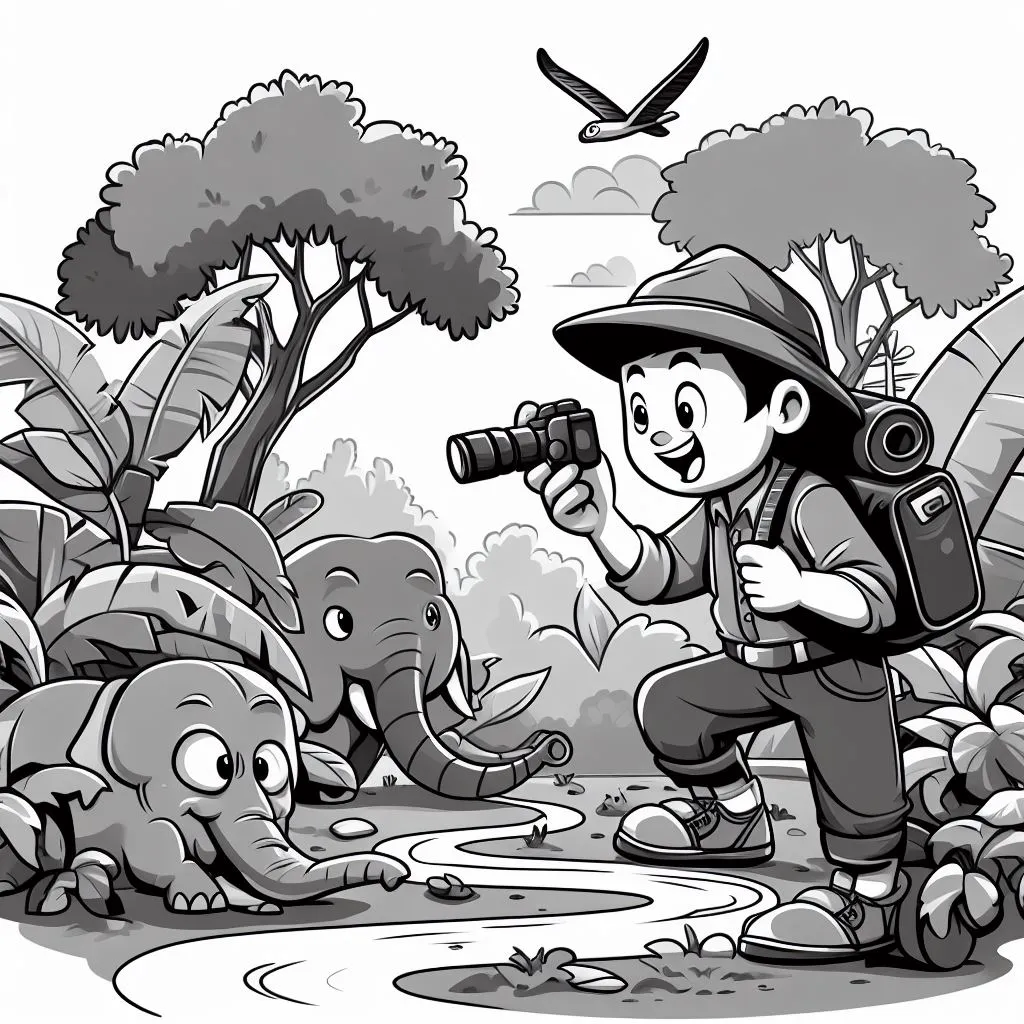
The Bayesian approach, in contrast to the frequentist approach, is a theory of probability that incorporates prior beliefs or knowledge about an event or experiment. It updates these prior beliefs using observed evidence to create a posterior probability distribution.
In a coin-toss experiment, the Bayesian approach considers not only the observed outcomes but also any prior information or beliefs we may have about the coin. Suppose we have a prior belief that the coin may not be fair, and we assign a certain probability distribution to the possible bias of the coin.
As we conduct the coin tosses and observe the outcomes, our prior beliefs are updated to form the posterior probability distribution. The observed data is used to revise our initial beliefs about the coin's bias. This updating process follows Bayes' theorem, which involves multiplying the prior probability by the likelihood of the observed data given that prior, and then normalizing to obtain the posterior probability distribution.
The Bayesian approach allows for the incorporation of subjective prior information and enables us to update our beliefs based on new evidence. It provides a framework to quantify uncertainty and make decisions incorporating both prior knowledge and observed data. Unlike the frequentist approach, which treats probability as the long-term frequency of an event, the Bayesian approach treats probability as a measure of subjective belief.
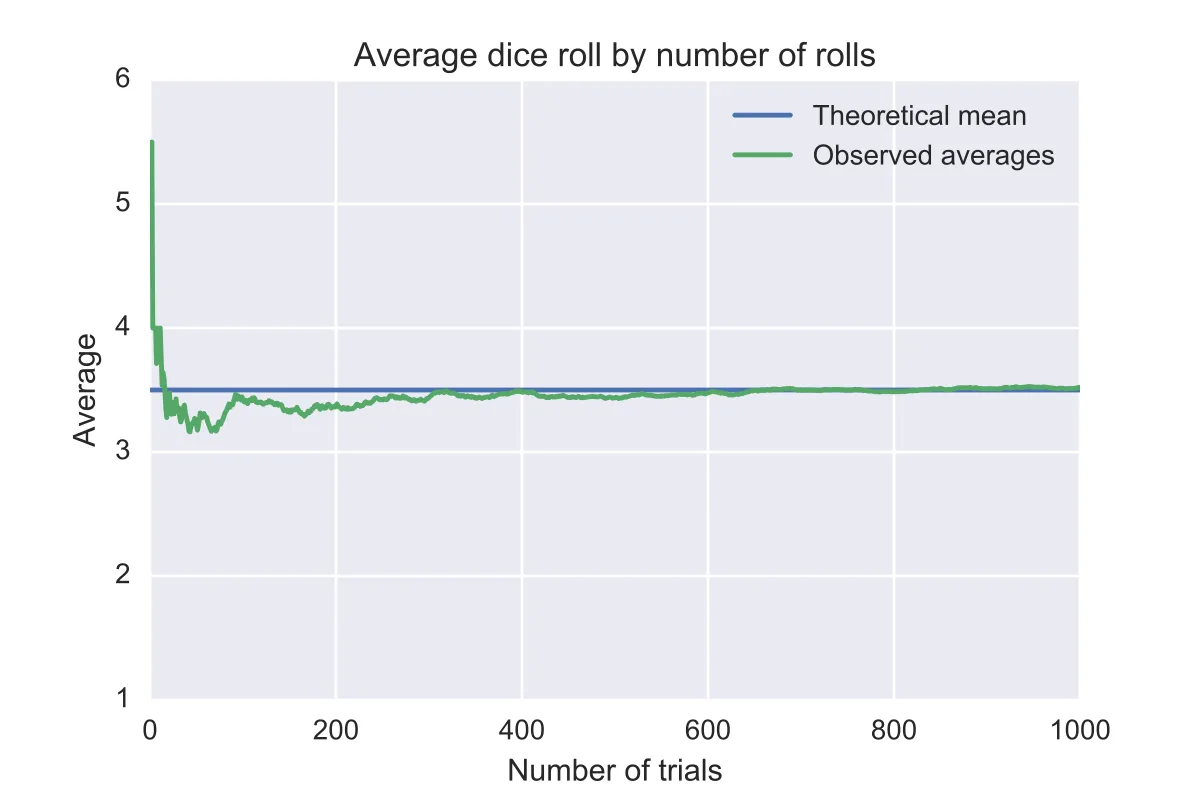
As you continue to toss the coin more, you keep updating your belief based on the observed results. After many more tosses, the Bayesian and Frequentist approaches would likely converge to similar conclusions which is 50% in a coin tossed experiment.
The Controversy Between Bayesian and Frequentist
The controversy between Bayesian and Frequentist statistics stems from their contrasting interpretations of probability.
Frequentists view probability as an objective measure of the limit of an event’s frequency after numerous repetitions. They argue for objectivity and repeatability in talks of probability and base their claims on observations. Critics note, however, this approach might fail to incorporate important contextual aspects in a complex scenario.
On the other hand, Bayesians see probability as a subjective measure of personal belief or confidence, which can change as more evidence is gathered. Critics argue this approach may become too subjective and dependent on the individual’s views, yet it allows for greater flexibility in incorporating prior knowledge and addressing complex scenarios.
The controversy between these two approaches also extends to decision-making processes in statistics, experimental design, and A/B testing.
Frequentists rely on null hypothesis significance testing, while Bayesians involve loss functions and expected loss, offering a more intuitive understanding of results.
Frequentist vs Bayesian in A/B Testing
Evaluating the results of an A/B test often involves the use of statistics, beginning with gathering data and calculating averages, and then progressing to inferential statistics.
Here, both Bayesian and Frequentist methods can be utilized to understand the true impact of an intervention or choice.
.webp)
Frequentist Role in A/B Testing
The hallmark of the Frequentist approach is the formulation of a null hypothesis that states there is no difference between treatments A and B. The goal is to provide evidence contrary to this hypothesis, based on a sample of data.
Consider a situation where you’re testing two landing pages, A and B, for your website. To deploy Frequentist methods, you would propose the null hypothesis that both pages generate the same number of clicks or interactions. You would then collect data on user interaction with each variant and use statistical methods like T-tests or Chi-square tests to calculate a p-value.
This p-value tells you the likelihood of obtaining the observed data if the null hypothesis were true. That is, assuming there is no difference between the landing pages. If such probability (p-value) is very small (usually less than 0.05), you may reject the null hypothesis and conclude that there is a difference between the two pages.
While it has the advantage of simplicity and objectivity, Frequentist methods do not take into account prior knowledge about the situation being tested. Also, they rely heavily on the idea of repetition under the same conditions, which might be hard to achieve in practice.
Bayesian Role in A/B Testing
The Bayesian approach brings a different perspective. Rather than looking for evidence against a null hypothesis, Bayesian statistics are about updating prior “beliefs” based on the collected data.
In the context of A/B testing, this would involve using data obtained from your A/B test to update a prior belief about the performance between two landing pages. This is done by observing the data (the number of clicks on both page A and page B) and adjusting your belief in light of this new evidence.
The result is a ‘posterior’ probability that represents your updated belief after considering the data. Since your belief is continuously updated as more data is collected, the Bayesian approach is often thought of as a dynamic process.
One of the strengths of this approach is its ability to integrate prior knowledge into the analysis and provide a probabilistic framework for expressing uncertainty. Bayesian methods can also deal with complex modeling and inference problems that are challenging for frequentist methods.
However, Bayesian methods can be computationally intensive, especially for complex problems with high-dimensional parameter spaces. Also, they require a prior which may be subjective and a potential source of controversy.
Both frequentist and Bayesian have their advantages and limitations but provide useful insights for conducting and interpreting A/B tests. Understanding these methods can help optimize and make more informed decisions based on the results of A/B testing.
Which Approach Should You Use?
The choice between Bayesian and Frequentist methodologies largely depends on your comfort level with each approach, the nature of your problem, and the availability of prior knowledge.
The key takeaway is understanding that different statistical methodologies exist, they offer different insights and definitions of probability. A/B testing and data interpretation is not a one-size-fits-all thing.
The important thing is to understand the statistical method you are using for A/B testing and how the conclusions are derived, because regardless of whether you’re a Bayesian or a Frequentist, data-driven decision-making is the way forward!